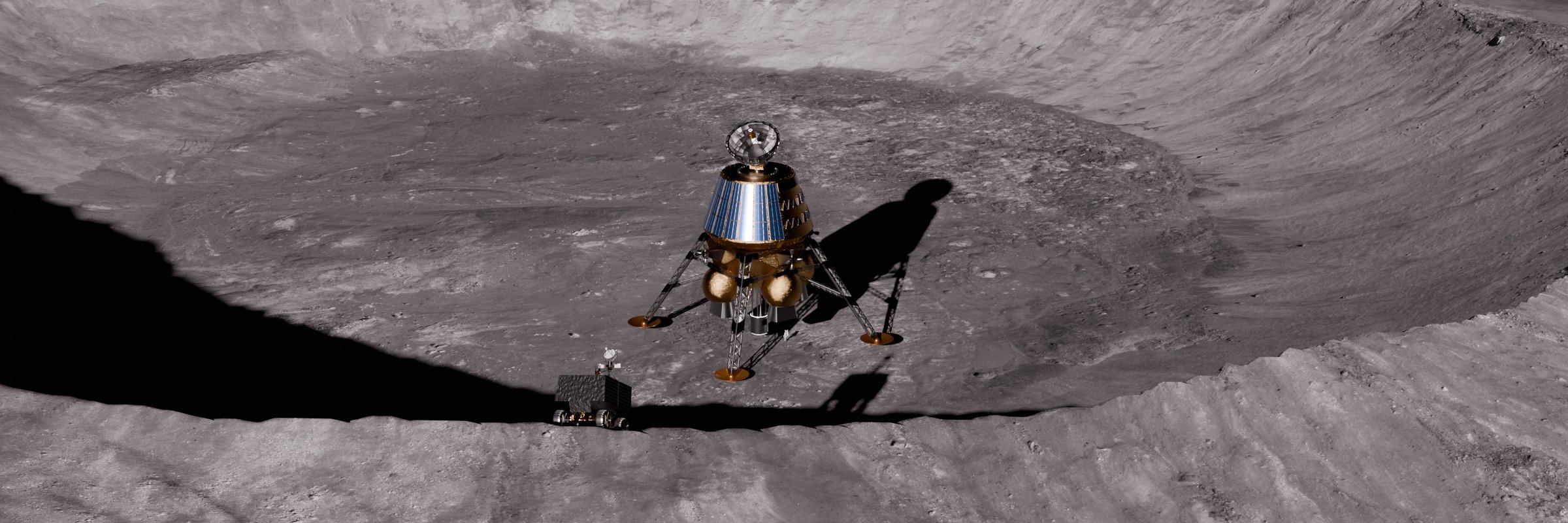
AI for lunar exploration
Over the past 7 years, NASA, The Luxembourg Space Agency / Space Resources and FDL.AI have had a hugely impactful research partnership leveraging AI for lunar exploration and resources, with notable firsts in advance of the Artemis mission.
The formula of data science specialists working closely with subject matter researchers has been remarkably consistent, with many peer reviewed research outcomes and data products, from rover localization without GPS to co-operative robotics and crater-counting for impact age determination. Some of our most impactful work for Artemis is shared below.
Notable firsts:
Lunarlab’s research used AI to peer into the permanently shadowed regions (PSRs) of the Moon to give us a clear view into the permanent darkness for the very first time. HORUS continues to be used for traverse planning.
Lunarlab’s work on thermal anomaly detection revealed the location of the Moon’s metallic resources using a breakthrough application of Physics Informed Neural Nets called a PINN. This AI allows us to see the Moon’s resources clearly.
Lunar South Pole PSRs
Supporting lunar exploration
CHANGE DETECTION WITH LRO DIVINER
We can use ML to identify the faint and transient signatures of features such as fresh craters, landslides, and tectonic activity on the whole Moon. Protecting the settlers and infrastructure of our off planet outpost.
UPSCALING LUNAR RESOURCES
We can use AI super-resolution to improve maps of the lunar poles upscaling intermediate (low res) imagery to high resolution images. The improved images have substantial value for lunar science, greatly aiding the traverse planning for the imminent exploration of the Moon.
MOON FOR GOOD (PHASE II)
ML can learn to enhance the light in faint images of permanently shadowed regions of craters, to aid sustainable missions on the Moon that depend on ice deposits.
LUNAR RESOURCE MAPPING / SUPER RESOLUTION
This team produced a global data stack of lunar orbiter measurements by fusing 42 layers of multi-sensor satellite data. Revealing the location of high value metallic deposits on the moon, enabling the construction of a sustainable off planet outpost on the moon.
2024
Superresolution of Lunar Satellite Images for Enhanced Robotic Traverse Planning: Maximizing the Value of Existing Data Products for Space Robotics - IEEE Robotics & Automation Magazine, June 2024
Automated Discovery of Anomalous Features in Ultralarge Planetary Remote-Sensing Datasets Using Variational Autoencoders - IEEE Journal of Selected Topics in Applied Earth Observations and Remote Sensing Volume 17, February 2024
2021
Peering into lunar permanently shadowed regions with deep learning - Nature Communications (FDL USA 2020), September 2021
Unsupervised Learning for Thermophysical Analysis on the Lunar Surface - The Planetary Science Journal (FDL USA 2021), July 2021
Absolute Localisation for surface robotics in GPS denied locations using a Neural Network- JPL AI and Data Science 2021 Workshop (FDL USA 2018)
2020
Thermophysical Change Detection on the Moon with the Lunar Reconnaissance Orbiter Diviner sensor - Machine Learning and the Physical Sciences workshop, NeurIPS 2022 (FDL USA 2020)
Low-light image enhancement of permanently shadowed lunar regions with physics-based machine learning - University of Luxembourg Library (FDL USA 2020), December 2020
Unsupervised learning for thermal anomaly detection on the lunar surface- 51st Lunar and Planetary Science Conference - 2020 (FDL USA 2019)
Unsupervised learning for thermal anomaly detection on the lunar surface - The Planetary Science Journal 2020 (FDL USA 2019), July 2020
Low-Light Image Enhancement of Permanently Shadowed Lunar Regions with Physics-Based Machine Learning - University of Luxembourg Library / CVPR conference - NVIDIA GTC21 - Accepted (FDL USA 2020)
A Big Data and AI-Driven Approach for Anomaly Detection on the Lunar Surface - Lunar and Planetary Institute (FDL USA 2020), March 2020
Absolute Localisation for surface robotics in GPS denied locations using a Neural Network - IEEE Xplore 2019 (FDL USA 2019), January 2020
2019
Absolute Localization Through Orbital Maps and Surface Perspective Imagery - 2019 IEEE/RSJ International Conference on Intelligent Robots and Systems (IROS) (FDL USA 2018) November 2019
Unsupervised learning for thermal anomaly detection on the lunar surface - NeurIPS Workshop 2019 (FDL USA 2019)
Unsupervised Distribution Learning for Lunar Anomaly Detection- NeurIPS 2019 (FDL USA 2019)